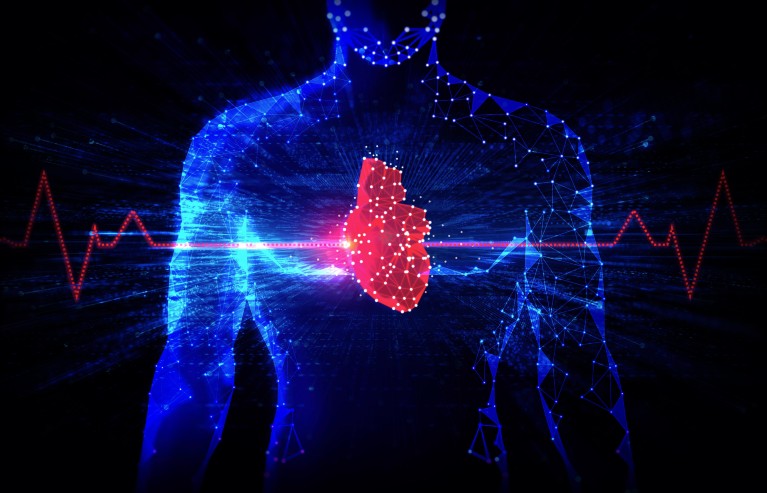
Credit: ArtemisDiana / Getty Images
Cardiovascular diseases (CVDs) are the leading cause of death worldwide. The level of danger is dependent on many risk factors, but doctors currently have only a handful of broad clinical indicators — such as blood pressure, cholesterol levels or diabetes — to inform a prognosis and direct patients to proper preventative care.
In April 2022, Stephen Williams, the chief medical officer at Boulder, Colorado-based biotech company SomaLogic, led a multi-national study, which demonstrated the power and promise of an alternative prognostic strategy. Researchers used a proteomics platform to identify and validate a set of biomarkers that collectively provide a window into a person’s heart health. The resulting panel of proteins outperformed existing clinical risk scores, and revealed a subset of ultra-high-risk patients with a greater than 43% chance of experiencing a cardiovascular event, such a heart attack or stroke, within the next four years (Williams, et al. Sci Tran Med 14, 2022).
A growing number of studies are highlighting how insights into the human proteome — the set of all proteins in the body — can guide diagnosis, treatment, and medical understanding of CVD. Armed with the right protein biomarkers, clinicians could soon have the tools they need to deliver highly patient-specific preventative and interventional care.
Signatures of heart health
Williams believes proteins are ideal biomarkers for assessing health. “Protein networks have evolved to transmit information from one biological system to another, and they're the targets of 95% of all known drugs,” he says. The information conveyed by proteins is informed by genetic factors that contribute to disease risk, but also influenced by lifestyle and environmental factors, providing a real-time indicator of a person’s physiological state.
Analysing the proteome can be challenging, however. One of the most widely used methods is mass spectrometry (MS), but this is not always ideal for clinical diagnostic and prognostic applications. Peter Ganz, a cardiologist at the University of California, San Francisco, and co-lead author on the proteomics study, points out that MS is slow and lacks the dynamic range for effective biomarker discovery. “If you do an untargeted experiment, you can measure proteins that are highly abundant, but you cannot measure proteins in low concentrations,” he says. “Many of the most clinically interesting proteins may be quite scarce in a sample.”
SomaLogic is tackling this problem by assembling libraries of ‘slow off-rate modified aptamers’, or SOMAmer® reagents. These chemically-modified, DNA-based molecules are selected and engineered to bind and remain tightly coupled to specific protein targets. SOMAmer reagents are applied to clinical samples in the context of the SomaScan® Assays, which can be used to simultaneously detect up to 7,000 different proteins spanning a dynamic range of ten orders of magnitude—from femtomolar to micromolar concentrations. And critically, the assay is much faster than MS. “We are doing population studies where we have thousands of individuals involved,” says Ganz. “With MS, it would take me a lifetime to try to analyse these — here, we can do it in a matter of weeks.”
Profiling patients’ proteins
Williams and Ganz’s proteomics study used the SomaScan Assay to analyse plasma samples from nearly 23,000 people. They applied machine learning to develop a risk-assessment model based on 27 protein biomarkers, which they validated with plasma samples from over 11,000 additional people. The resulting model proved broadly applicable across people of different sex, race and national origin. This is not merely a static snapshot of risk, however; the scoring strategy was also responsive to changes in lifestyle and medical treatment, reflecting the resulting improvements or declines in apparent cardiovascular health.
Williams is especially enthusiastic about this latter aspect of the work, which highlights the potential of using this protein panel as a ‘surrogate marker’ for studying drug response in clinical trials: to see whether a patient’s disease is improving or worsening. “In oncology, there are surrogate endpoints like progression-free survival used early in development to get rid of the drugs that don't work,” he says. “In CVD, you have to wait for outcomes.” This makes such trials slow and expensive. SomaLogic is working with the US Food and Drug Administration to pursue regulatory authorization for the use of its assay as an outcome measure.
The SomaScan Assay is also being used to tackle questions related to specific subtypes of CVD. For example, in 2020, cardiologist, Ali Javaheri, at Washington University in St Louis used this platform to confirm that a protein called apolipoprotein M plays a prominent role in protecting against heart failure, and identified a molecular pathway by which it may exert this effect. And Yuichi Shimada, a CVD specialist at Columbia University in New York City, used this proteomics strategy to uncover biological networks involved in hypertrophic cardiomyopathy (HCM), a genetic disorder that puts young people at risk of sudden cardiac death. “We elucidated that one specific signalling pathway, Ras-MAP, is upregulated in HCM, and has prognostic value in terms of predicting future cardiovascular events,” says Shimada.
These analyses can also reveal subtle but clinically important subcategories of disease. Javaheri notes that heart-failure patients are often diagnosed based on left ventricular ejection fraction, which measures how much blood is pumped out of the heart per beat. But this doesn’t catch every patient. “Proteomics gives you a way of phenotyping the patient that's different and probably higher resolution,” says Javeheri. Shimada is seeing similar benefits in using proteomic analysis to classify HCM patients and guide individualized treatment; his team has found one HCM subtype that is specifically associated with overactivation of the Ras-MAPK pathway. “There is a specific inhibitor of this Ras-MAPK pathway already available,” says Shimada, “this could translate to an effective targeted therapeutic for certain patients.”
Room to grow
This is only the beginning for clinical proteomics. The next iteration of the SomaScan platform — due for release in 2023 — will encompass 10,000 proteins, but still represents less than half of the full human proteome. Williams points out that as the assay has grown, it has continued to gain predictive power. “As we've added more proteins, our machine learning platform is just as likely to choose them as being the most informative proteins,” he says.
Broader proteomic coverage with a unified assay will also make it easier to study the interaction of CVDs with associated conditions like diabetes or chronic kidney disease. “From a single blood sample,” says Ganz, “one can simultaneously determine the risk of many diseases.”